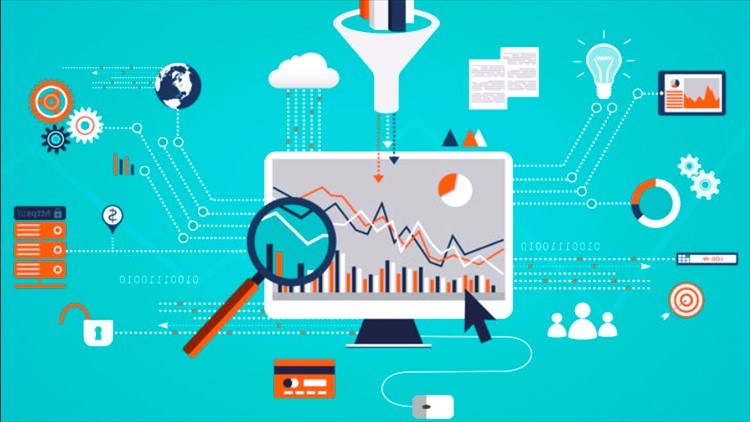
The Role of Data Analytics in Optimizing Production Testing Processes
BlogIn the era of Industry 4.0, where connectivity and data-driven decision-making are at the forefront of technological advancements, the integration of data analytics into production testing processes is transforming the landscape of quality assurance. This article explores the pivotal role of data analytics in optimizing production testing, shedding light on how leveraging data insights can enhance efficiency, accuracy, and overall effectiveness in manufacturing.
1. The Evolution of Production Testing:
Production testing has evolved from traditional manual methods to sophisticated, automated processes that generate vast amounts of data. While the primary goal remains ensuring product quality, the integration of data analytics introduces a paradigm shift by offering a deeper understanding of testing processes and outcomes.
2. Harnessing Big Data for Testing Insights:
The sheer volume and complexity of data generated during production testing necessitate advanced analytical tools and methodologies. Big Data analytics allows manufacturers to process, analyze, and derive actionable insights from large datasets. This includes data from sensors, testing equipment, and other connected devices throughout the production process.
3. Improved Testing Accuracy and Predictability:
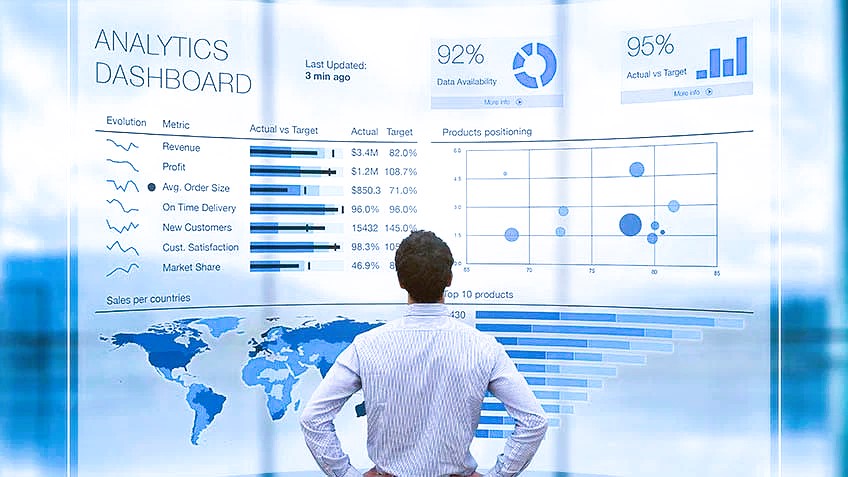
Data analytics contributes to improved testing accuracy by identifying patterns and anomalies in testing outcomes. Through machine learning algorithms, analytics can predict potential issues, enabling proactive measures to be taken before defects occur. This level of predictability enhances overall testing precision and reduces the likelihood of defects reaching the final product.
4. Real-Time Monitoring and Decision-Making:
The integration of data analytics enables real-time monitoring of production testing processes. This means that manufacturers can receive instant feedback on testing outcomes, allowing for immediate decision-making. Rapid response to testing data ensures that adjustments can be made promptly, minimizing the impact of issues and optimizing the overall manufacturing process. Did you like the article? Read also about Vibration Testing.
5. Identifying Root Causes of Defects:
When defects are identified during production testing, data analytics plays a crucial role in root cause analysis. By analyzing historical data and correlating it with specific defects, manufacturers can pinpoint the root causes of issues. This insight is invaluable for implementing targeted corrective actions and preventing the recurrence of similar defects in future testing cycles.
6. Enhancing Equipment Utilization and Maintenance:
Data analytics contributes to the optimization of testing equipment utilization. By analyzing equipment performance data, manufacturers can identify usage patterns, predict maintenance needs, and optimize the scheduling of equipment downtime. This proactive approach minimizes unplanned equipment failures, ensuring consistent testing capabilities.
7. Streamlining Testing Workflows:
Understanding the flow of testing processes is essential for efficiency. Data analytics provides insights into testing workflows by identifying bottlenecks, unnecessary delays, or redundant steps. By streamlining testing workflows based on data-driven insights, manufacturers can achieve higher throughput, reduce testing cycle times, and enhance overall operational efficiency.
8. Data-Driven Continuous Improvement:
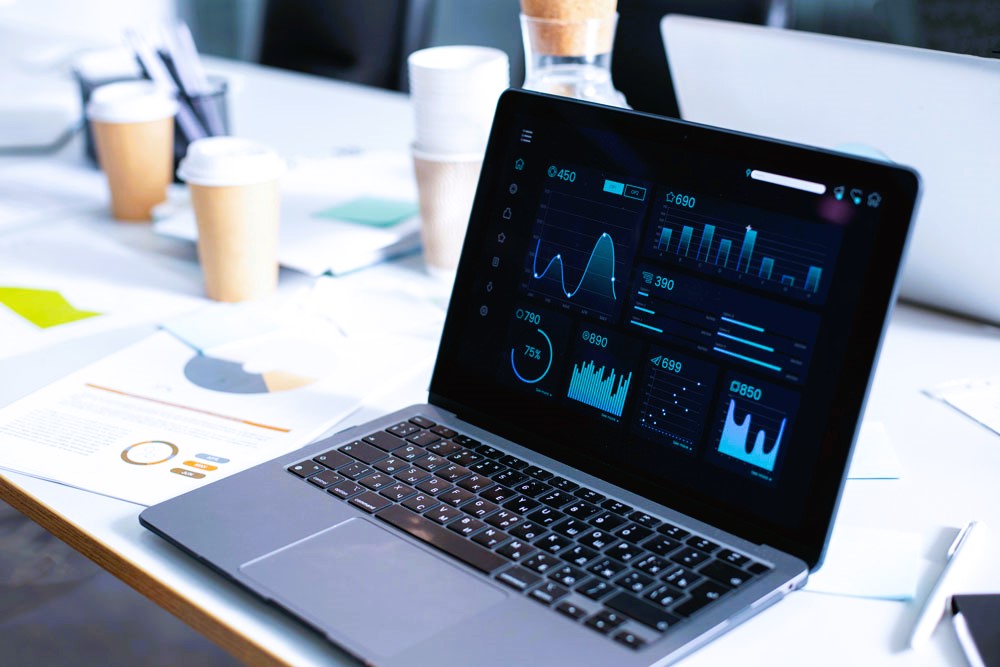
One of the key benefits of integrating data analytics into production testing is its role in driving continuous improvement. By analyzing historical testing data, manufacturers can identify trends, assess the effectiveness of process changes, and iteratively improve testing methodologies. This data-driven continuous improvement cycle ensures that testing processes evolve in tandem with industry advancements and changing product requirements.
Linking to Standards:
The integration of data analytics into production testing aligns with industry standards that emphasize the importance of leveraging technology to enhance quality assurance processes. Standards provide a framework for incorporating data-driven approaches into testing methodologies, ensuring that manufacturers adhere to best practices in utilizing analytics for optimization. For more information on standards in Canada, you can refer to authoritative sources such as Canada.ca.
Conclusion: Navigating the Future of Quality Assurance
In the ever-evolving landscape of manufacturing, data analytics emerges as a powerful ally in the quest for optimized production testing processes. The ability to harness insights from big data not only enhances testing accuracy and predictability but also enables manufacturers to make real-time decisions, identify root causes of defects, and continuously improve testing workflows.
As manufacturing processes become increasingly interconnected, the role of data analytics becomes even more pivotal. By leveraging the wealth of data generated during production testing, manufacturers can navigate the complexities of modern production environments, ensuring that the products reaching the market meet the highest standards of quality and reliability.
The integration of data analytics into production testing is not merely a technological enhancement; it is a strategic imperative for manufacturers aiming to stay competitive in a data-driven era. The insights derived from analytics empower manufacturers to optimize testing processes, reduce costs, and, most importantly, deliver products that meet and exceed customer expectations. As technology continues to advance, the role of data analytics in production testing will undoubtedly shape the future landscape of quality assurance in manufacturing.
Recent Posts
- The Role of Data Analytics in Optimizing Production Testing Processes
- Measuring Success: Key Performance Indicators for Production Testing
- The Interplay Between Production Testing and Continuous Improvement
- The Importance of Training and Skill Development in Production Testing Teams
- Strategies for Minimizing Downtime During Production Testing